Why You Should Monitor Your AI Applications (Part 1)
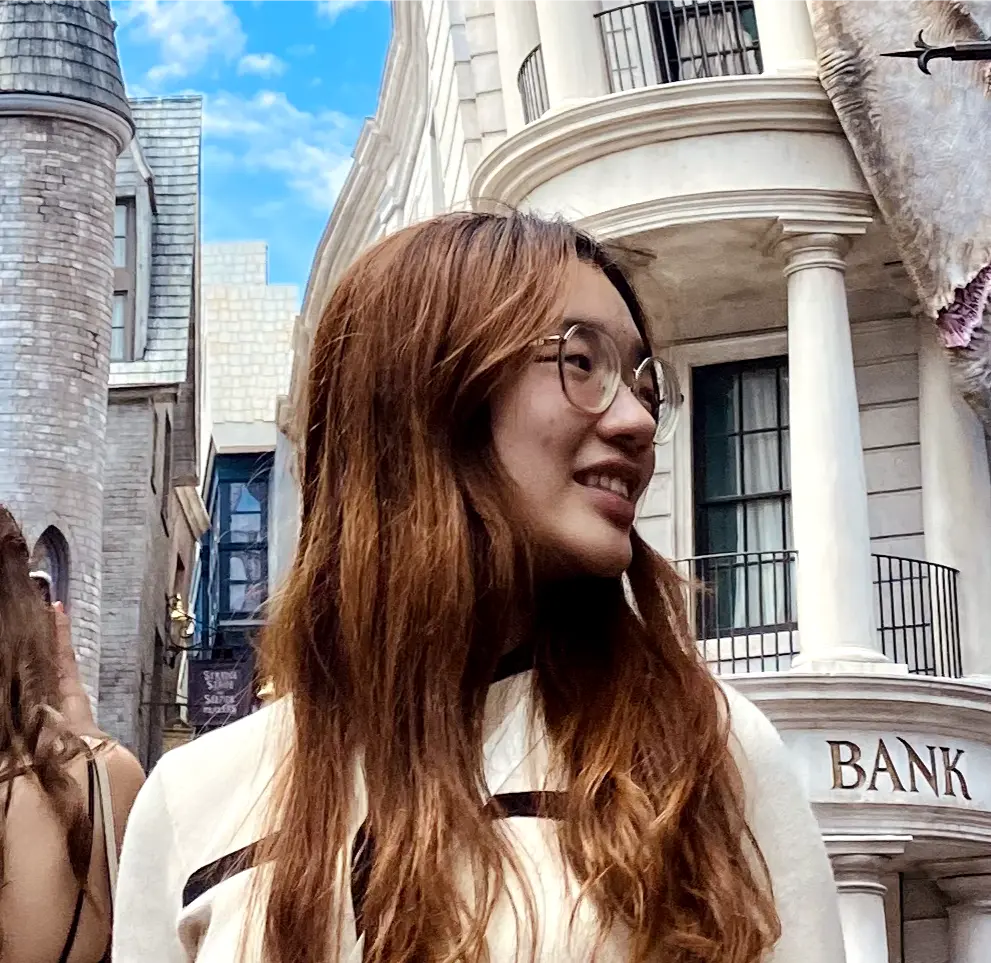
Today's AI applications are customer-facing, handle sensitive information, make important decisions, and quite frankly, represent your brand. At Helicone, we believe that effective monitoring is now a competitive advantage.
With tools like v0 and cursor, it's easier than ever to spin up an LLM app, but it's harder than ever to build reliable and production-ready LLM applications.
In this first guide of our two-part series, we'll explore:
- What is LLM observability?
- How it's different from traditional observability
- The key metrics you should be tracking
- The five pillars of comprehensive LLM monitoring
- Why observability is no longer optional
Let's dive in!
What is LLM Observability?
LLM observability refers to the comprehensive monitoring, tracing, and analysis of AI-powered applications. It involves looking into every aspect of the system, from prompt engineering, to monitoring model responses, to testing prompts and evaluating the LLM outputs.
Unlike traditional software where you can trace through deterministic code, LLMs operate as "black boxes" with billions of parameters, making observability critical for:
- Understanding how changes impact outputs: When you modify a prompt or switch models, how does that affect results?
- Pinpointing and debugging errors: Did your prompt change regress? Identify hallucination, anomalies, security issues or performance bottlenecks.
- Optimizing for cost and performance: Balance token usage, latency, and output quality.
The UX Benefits of LLM Observability 💡
With proper LLM observability, you'll catch and fix response delays and hallucinations before your users experience them. Nothing kills user trust faster than an agent that suddenly breaks or takes forever to respond. And with request tracing, you can quickly pinpoint issues instead of wasting hours on debugging.
The Business Benefits of LLM Observability
Beyond just technical understanding, LLM observability also has tangible business benefits, such as:
- Reducing operational costs by identifying expensive patterns and optimizing accordingly
- Improving user retention by detecting and fixing poor experiences before they impact users
- Accelerating development cycles by iterating on prompts and debugging faster
- Increasing compliance by maintaining audit trails for regulated industries
- Justifying AI investment by showing clear ROI and performance metrics to stakeholders
As you build your product from prototype to production, monitoring LLM metrics helps you to detect prompt injections, hallucinations and poor user experience, allowing you to improve your prompts for better performance on the go.
LLM Observability vs. Traditional Observability
LLMs are highly complex and contain billions of parameters, making it challenging to understand how prompt changes affect the model's behavior.
While traditional observability like Datadog focuses on system logs and performance metrics, LLM observability deals with model inputs/outputs, prompts, and embeddings.
Another difference is the non-deterministic nature of LLMs. Traditional systems are often deterministic with expected behaviors, whereas LLMs frequently produce variable outputs, making evaluation more nuanced.
In summary:
Traditional Observability | LLM Observability | |
---|---|---|
Data Types | System logs, performance metrics | Model inputs/outputs, prompts, embeddings, agentic interactions |
Predictability | Deterministic with expected behaviors | Non-deterministic with variable outputs |
Interaction Scope | Single requests/responses | Complex conversations that can be multi-step, contains context over time |
Evaluation | Error rates, exceptions, latency | Error rate, cost, latency, but also response quality and user satisfaction |
Tooling | APMs, log aggregators, monitoring dashboards like Datadog | Specialized tools for model monitoring and prompt analysis like Helicone |
The Pillars of LLM Observability
1. Request and Response Logging
At the core of LLM observability is logging. When you log requests and responses, you can analyze patterns easier and understand whether model outputs meet user expectations.
Here are some data points you can capture:
Metric | Description |
---|---|
Input Prompt | The exact prompt sent to the model. |
Output Completion | The response generated by the model. |
Metadata | User IDs, session information, timestamps, and custom properties |
Cost | How much does it cost to generate a response? |
Performance metrics | Token counts, Time to First Token (TTFT), etc. |
Example
// With Helicone proxy integration
const openai = new OpenAI({
apiKey: process.env.OPENAI_API_KEY,
baseURL: "https://oai.helicone.ai/v1",
defaultHeaders: {
"Helicone-Auth": `Bearer ${process.env.HELICONE_API_KEY}`,
"Helicone-User-Id": userId,
"Helicone-Session-Id": sessionId,
"Helicone-Session-Path": "/abstract",
"Helicone-Session-Name": "Course Plan",
"Helicone-Property-App-Version": "v1.2.3",
"Helicone-Property-Feature-Flag": "experiment-12"
}
});
2. Tracing Multi-Step Workflows
Once you log requests and responses, and have a baseline of metrics, the next focus is optimizing the LLM responses and debugging issues.
With your baseline metrics established through request and response logging, the next step is gaining visibility into complex interactions between components.
Tracing allows you to:
- Follow conversation threads and agent interactions from start to finish
- Identify bottlenecks or failures in multi-step reasoning chains
- Visualize how different components interact across your app
- Diagnose exactly where and why errors occur
Unlike traditional applications, LLM systems often involve multiple reasoning steps, tool calls, and nested workflows that are difficult to debug without proper tracing capabilities.
Tools like Helicone's Sessions allows you to visualize workflows easily. You can use Sessions to pinpoint the exact request that caused the issue and debug faster. We've curated some useful examples how to debug agents with Sessions.
Here's an example 💡
When troubleshooting slow responses, Sessions lets you see exactly where delays occur - whether in the initial prompt processing, token generation, or a specific step in your agent workflow. This visibility helps you target optimizations precisely where they'll have the most impact.
3. LLM Evaluation
Evaluating LLM output quality is vital for continuous improvement. You need to evaluate quality both online (during production) and offline (post-production):
- Human feedback - Direct user ratings (i.e. thumbs up/down)
- Model-based evaluation - Using specialized models to assess outputs (i.e. LLM-as-a-judge)
- Reference-based metrics - Comparing responses against benchmarks
- Ground truth comparison - Automated comparison against known correct answers
- Automated scoring - Algorithmic assessment of coherence, accuracy, and relevance
It's good to have a mixture of methods. Collecting user feedback directly can give you valuable insights, but automated evaluation methods can give you consistent assessments when human evaluation isn't practical.
This pillar helps you quantify performance, detect regressions, and make data-driven decisions about model and prompt improvements.
4. Anomaly Detection and Feedback Loops
Detecting anomalies, like unusual model behaviors or outputs indicating hallucinations or biases, is essential for maintaining application integrity.
Common anomalies to watch for include:
- Statistical outliers - Responses significantly longer/shorter than normal
- Confidence scores - Unusually low confidence in generated answers
- Semantic drift - Outputs that deviate from expected topics or tone
- Potentially harmful content - Toxic, biased, or unsafe outputs
- Unusual patterns - Sudden changes in user behavior or model performance
Implementing mechanisms to scan for inappropriate or non-compliant content helps prevent ethical issues. Feedback loops, where users can provide input on responses, facilitate iterative improvement over time.
5. Security and Compliance
Securing your LLM applications requires a multi-layered approach that addresses both common web security concerns and LLM-specific threats. For example, implementing strict access controls to regulate who can interact with model inputs and outputs.
Key LLM security concerns include:
- Prompt injection attacks - Malicious inputs designed to manipulate model behavior
- Data leakage - Inadvertent exposure of sensitive information in outputs
- Hallucination risks - Incorrect or fabricated information presented as factual
- Content safety - Preventing generation of harmful, toxic, or inappropriate outputs
- Compliance traceability - Maintaining audit trails for regulatory requirements
Helicone provides built-in security capabilities that can be enabled with a simple header. For example:
import { Configuration, OpenAIApi } from "openai";
const configuration = new Configuration({
apiKey: process.env.OPENAI_API_KEY,
basePath: "https://oai.helicone.ai/v1",
defaultHeaders: {
"Helicone-LLM-Security-Enabled": "true",
"Helicone-LLM-Security-Advanced": "true",
},
});
const openai = new OpenAIApi(configuration);
For regulated industries, it's useful to implement additional guardrails such as PII detection, content filtering, and comprehensive logging. These protections not only safeguard your application but also helps to meet compliance requirements for GDPR, HIPAA, and emerging AI regulations.
It's all about building user trust!
We think that observability is no longer optional
The LLM space is evolving so fast. Effective observability was a nice-to-have, but it's becoming essential for any production application. Here's why:
- LLMs aren't static - Models are continuously updated, and what works today might not work tomorrow
- Costs add up quickly - Without monitoring, you may be spending far more than necessary
- User expectations are rising - As LLM applications become commonplace, users expect higher quality and reliability
- Competitors are watching - Companies with better observability can iterate faster and deliver superior experiences
- Compliance is coming - Regulations around AI transparency and safety are increasing
Coming next: Implementation
In our next guide How to Implement LLM Observability for Production (Part 2), we'll dive into:
- Best practices for monitoring LLM performance
- Code examples for implementing each observability pillar
- Step-by-step guide to getting started with Helicone
- Practical next steps for your LLM application
Keep reading to see how we'll turn these concepts into concrete actions.
You might find these useful:
- 5 Powerful Techniques to Slash Your LLM Costs
- Debugging Chatbots and LLM Workflows using Sessions
- How to Test Your LLM Prompts (with Helicone)
Questions or feedback?
Are the information out of date? Please raise an issue or contact us, we'd love to hear from you!